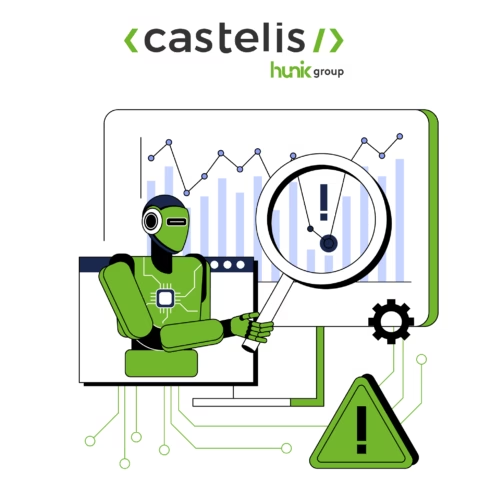
Optimizing cybersecurity threat detection
Every day, millions of cyberattacks are detected… but how many go unnoticed?
In the face of increasingly sophisticated attacks, companies must go beyond traditional antivirus and firewalls. The key? Advanced tools capable of detecting, analyzing, and neutralizing threats in real time.
💡 How can this detection be improved?
AI and Machine Learning today enable:
- analyzing massive volumes of data,
- spotting suspicious behaviors,
- automating incident response.
Microsoft Sentinel is one of the most powerful solutions, utilizing AI to correlate events and detect attacks upstream. Other tools like Splunk, Elastic Security, Darktrace, or IBM QRadar also provide effective approaches tailored to the needs of each organization.
- Which cybersecurity tools should you choose?
- How to optimize their use for maximum protection?
In this article, we will explore the best strategies and technologies to strengthen your cybersecurity posture.
Understanding Cyber Threat Detection
What is Cyber Threat Detection?
Threat detection involves identifying, analyzing, and responding to cyberattacks in real time. Its goal is to prevent or minimize the damage caused by attacks before they compromise systems.
There are two approaches:
- Preventive Detection: Anticipating threats before they occur using behavioral analysis and predictive models.
- Reactive Detection: Identifying an ongoing attack or one that has already been triggered to limit its impact.
Why is this crucial?
A delay in detection can lead to:
- Data theft: Notable examples at Equifax and Yahoo.
- Infrastructure paralysis: Cases like WannaCry and NotPetya ransomware attacks.
- Financial loss and reputational damage: Direct impact on targeted companies, especially in the banking and public sectors.
Effective detection is the first line of defense against these threats.
Have questions about threat detection? Fill out our form to get more information and personalized support.
Current Challenges in Cyber Threat Detection
1. The explosion of data volumes to analyze
With the rise of Big Data and the cloud, companies must process millions of security events per day. Real-time analysis has become a major challenge, requiring solutions that can filter relevant alerts without overwhelming SOC (Security Operations Center) teams.
2. A growing diversity of cyber threats
Attacks are rapidly evolving and taking many forms:
- Ransomware: File encryption and ransom demand.
- Phishing: Credential theft via fraudulent emails.
- Zero-day attacks: Exploiting vulnerabilities before vendors release patches.
3. Limitations of traditional security solutions
Traditional cybersecurity tools are no longer sufficient against new threats:
- Insufficient antivirus & firewalls against advanced attacks.
- Alert overload and false positives, slowing down team responses.
- Lack of global visibility: Fragmented solutions making real-time incident analysis difficult.
4. AI and Automation: Essential Allies
To address these challenges, companies are turning to artificial intelligence and automation, which offer:
- Intelligent correlation of suspicious events to reduce false positives.
- Advanced behavioral analysis detecting anomalies in real-time.
- Automated responses allowing threats to be isolated or neutralized within seconds.
In the face of these challenges, AI and machine learning have become essential pillars to improve threat detection efficiency and reduce risks associated with cyberattacks.
Role of AI and Machine Learning in Threat Detection
Artificial Intelligence (AI) and Machine Learning (ML) are revolutionizing cybersecurity by enabling the analysis of massive amounts of data, detecting threats in real-time, and automating responses to attacks. With these technologies, businesses can improve their ability to detect cyber threats while reducing the workload of IT security teams.
Benefits of AI and Machine Learning
Anomaly Detection and Behavioral Analysis
Traditional cybersecurity approaches rely on known signature-based databases, making them ineffective against new and unknown threats. AI, on the other hand, analyzes user, endpoint, and network traffic behaviors to identify suspicious anomalies.
- For example, an employee suddenly accessing sensitive files outside their normal working hours can trigger an alert.
- AI can also detect unusual network activity, signaling an ongoing attack, such as data exfiltration or an attacker’s lateral movement within a network.
Reducing False Positives through Continuous Learning
One of the major challenges with traditional threat detection tools is the large number of false positives, which overwhelm security teams. AI and ML continuously learn from past incidents to refine detection rules and distinguish real threats from legitimate activities.
- A machine learning-based solution can understand that a user suddenly changing their IP address but following their usual browsing patterns is not necessarily a threat.
- This helps prevent unnecessary alerts from overwhelming cybersecurity analysts.
Automating Responses to Threats (SOAR – Security Orchestration, Automation, and Response)
AI is not just limited to detection; it also plays a key role in automating incident response through SOAR platforms.
- If an attack is detected, the system can automatically isolate an infected machine, block a compromised account, or alert security teams with a detailed report.
- This allows for a response in seconds, much faster than a human intervention.
Successful Applications of AI in Cybersecurity
1. Predictive Analysis to Anticipate Cyberattacks
With AI, it is possible to analyze attack patterns and trends to anticipate threats before they occur.
- A SIEM like Microsoft Sentinel uses AI to identify weak indicators of impending attacks, such as an abnormal increase in login attempts on a critical server.
- A machine learning-based solution can detect unusual behaviors and predict that a ransomware attack is spreading before it even encrypts files.
2. Detection of Suspicious Behaviors in Network Traffic
Companies using tools like Vectra AI or Cisco Secure Network Analytics can monitor their networks in real-time to identify anomalies that could indicate an attack.
- AI can detect an abnormally high rate of outgoing connections on a server, suggesting data theft.
- AI can also detect internal attacks, such as a malicious employee attempting to access confidential files.
AI and Machine Learning have become essential tools for detecting and responding to cyber threats. With their ability to analyze real-time behaviors, reduce false positives, and automate responses, these technologies allow companies to react more quickly and effectively to cyberattacks.
In the following sections, we will explore Microsoft Sentinel and other advanced solutions that integrate AI for optimized cybersecurity.
Introducing Microsoft Sentinel, a Cloud-Based SIEM
Main Features of Microsoft Sentinel SIEM
Microsoft Sentinel is a cloud-native SIEM (Security Information and Event Management) and SOAR (Security Orchestration, Automation and Response) solution designed to provide advanced protection against cyber threats. Hosted on Microsoft Azure, it allows you to collect, analyze, and correlate security logs in real-time to identify and neutralize threats effectively.
Log Collection and Analysis from Various Sources
- Microsoft Sentinel integrates with over 100 data sources, including of course Microsoft solutions (Azure, Microsoft Defender, Office 365) as well as third-party tools like Palo Alto Networks, Cisco, AWS, Google Cloud, and Splunk.
- It allows for aggregating event logs, network traffic, authentication logs, security alerts, and other essential data for threat analysis.
Real-Time Threat Correlation and Analysis
- Sentinel uses artificial intelligence and machine learning to detect attack patterns and identify anomalies before they cause damage.
- KQL (Kusto Query Language) queries enable SOC analysts to perform advanced analysis and identify suspicious trends.
Automating Alerts and Incident Responses
- With its SOAR engine, Microsoft Sentinel allows for automated incident responses with playbooks, reducing the workload of cybersecurity teams.
- It can automatically block malicious IP addresses, disable a compromised account, or isolate an infected machine by integrating with tools like Microsoft Defender XDR and Azure Logic Apps.
Specific Benefits of Sentinel for Cyber Threat Detection
1. Integration with Other Cybersecurity Tools
Microsoft Sentinel natively integrates with the Microsoft ecosystem, enabling centralized and seamless threat monitoring.
- Compatibility with Microsoft Defender XDR: Enhances endpoint, identity, and email protection.
- Integration with Azure Security Center: Provides a consolidated view of vulnerabilities and risks across the entire cloud and hybrid infrastructure.
- Integration with third-party solutions: Sentinel can be paired with AWS, Google Cloud, Palo Alto Networks, Cisco, Splunk, and more to ensure comprehensive threat coverage.
2. Advanced Event Correlation and Proactive Detection
- Sentinel combines multiple data sources to correlate events and detect complex attacks that would go unnoticed in a traditional SIEM.
- It uses AI-based detection models to reduce false positives and prioritize critical alerts.
- The “Hunting” feature allows analysts to search for hidden threats using predefined or customized queries.
3. Interactive Dashboard and Centralized Incident Management
- Sentinel offers an intuitive visual interface that allows for real-time tracking of the organization’s cybersecurity status.
- The centralized dashboard provides:
- A global view of active threats and ongoing incidents.
- Access to attack details, with interactive graphs and timelines.
- The ability to filter alerts to prioritize critical incidents and avoid overwhelming SOC analysts.
Why Choose Microsoft Sentinel?
Microsoft Sentinel is a powerful, scalable, and intelligent cloud-native SIEM, ideal for businesses looking to centralize threat management, automate incident response, and leverage AI to enhance their cybersecurity.
Disclaimer: A Choice Aligned with Our Expertise
At Castelis, we are a Microsoft partner and support many organizations in optimizing their IT security with Microsoft Sentinel and other Microsoft solutions. As our client base is largely under Microsoft environments, Sentinel often emerges as the ideal solution, as it integrates natively with Microsoft 365, Azure, and Defender XDR.
However, Microsoft Sentinel is not always the best option, and it’s important to evaluate each specific need before choosing a SIEM.
When Microsoft Sentinel is Not the Most Suitable Solution
- Enterprise seeking an on-premise SIEM solution → Sentinel is 100% cloud-based, making it unsuitable for organizations with policies prohibiting cloud use for sensitive logs. IBM QRadar or Splunk Enterprise might be better alternatives.
- Multi-cloud infrastructure without a Microsoft predominance → While Sentinel can integrate with AWS, Google Cloud, and other solutions, it works optimally in an Azure environment. For a truly agnostic approach, Splunk or Elastic Security may offer more flexibility.
- Organization requiring advanced network traffic detection (NDR) → Sentinel is a SIEM, not an NDR (Network Detection & Response). If real-time network threat monitoring is a priority, solutions like Darktrace or Vectra AI may be more appropriate.
- Limited budget and need for an open-source solution → Sentinel operates on a consumption-based log pricing model, which can become expensive at scale. An open-source alternative like Elastic Security may be more affordable for some companies.
Comparison with Other Solutions
While Sentinel is a top choice for businesses using Microsoft, other SIEM & XDR solutions exist, each with its strengths and limitations.
Other AI and Machine Learning Tools for Cybersecurity
While Microsoft Sentinel is a powerful cloud-native SIEM solution, it’s not always the best choice depending on the environment and specific business needs. Other solutions leveraging artificial intelligence and machine learning offer interesting alternatives, with varying features based on use cases.
Here’s an analysis of major cybersecurity tools integrating AI to improve threat detection and response:
Splunk Enterprise Security (Advanced SIEM & SOAR)
Overview
Splunk Enterprise Security is a robust SIEM that analyzes billions of logs in real-time to identify threats and provide a centralized view of security incidents. It also has SOAR capabilities to automate responses to attacks.
✅ Strengths
- Advanced analytics engine capable of processing large data volumes.
- Excellent event correlation to detect complex threats.
- Extensive integration with numerous cybersecurity tools.
⚠️ Limitations
- High cost, especially for large infrastructures handling large log volumes.
- Steep learning curve for security teams.
🔗 More info: Splunk Enterprise Security
Elastic Security (Open-source SIEM based on ELK)
Overview
Elastic Security is based on the Elastic Stack (ELK), an open-source solution specialized in log management and real-time threat detection. It offers great flexibility and can be deployed on-premise or in the cloud.
✅ Strengths
- Open-source model offering an economical alternative to commercial SIEMs.
- Flexibility and advanced customization with Kibana dashboards and Elasticsearch queries.
- Good threat detection capacity via machine learning.
⚠️ Limitations
- Requires technical expertise for configuration and maintenance.
- Less native automation compared to solutions like Sentinel or Splunk.
🔗 More info: Elastic Security
Darktrace (Behavioral Detection Powered by AI)
Overview
Darktrace uses machine learning to detect abnormal behaviors across networks, endpoints, and the cloud. It’s designed to identify unknown threats before they cause damage.
✅ Strengths
- Advanced behavioral analytics: identifies anomalies in real-time.
- Self-learning: improves detection accuracy over time.
- Autonomous response capability with its Antigena module.
⚠️ Limitations
- Many false positives, requiring fine-tuning of detection rules.
- Expensive solution, often suited for large enterprises.
🔗 More info: Darktrace
IBM QRadar (SIEM with AI-powered Event Correlation)
Overview
IBM QRadar is a SIEM known for its ability to deeply analyze security events and correlate threats at scale. It integrates with IBM Watson AI to enhance incident detection and analysis.
✅ Strengths
- Powerful correlation of threats with AI.
- Good integration with other SIEM and XDR tools.
- Can handle large log volumes with stable performance.
⚠️ Limitations
- Complex deployment and management, requiring specific expertise.
- High cost, especially for complete licenses with SOAR.
🔗 More info: IBM QRadar
Vectra AI (Specialized in NDR – Network Detection & Response)
Overview
Vectra AI is a solution specialized in network threat detection using deep learning and data flow analysis. It’s particularly suited for internal attacks and lateral movements.
✅ Strengths
- Real-time network monitoring to detect threats before data exfiltration.
- Detection of internal threats and identity compromises. Behavioral analytics powered by AI to reduce false positives.
⚠️ Limitations
- Must be coupled with a SIEM for complete incident management.
- Less effective in a 100% cloud environment without internal network traffic.
🔗 More info: Vectra AI
Cortex XDR (Palo Alto Networks) (Multi-vector Threat Management)
Overview
Cortex XDR, developed by Palo Alto Networks, is an XDR platform that correlates alerts from endpoints, network, and cloud to identify sophisticated threats.
✅ Strengths
- Multi-vector threat correlation (endpoints, network, cloud).
- Advanced automation with integrated SOAR playbooks.
- Excellent integration with Palo Alto’s firewalls and solutions.
⚠️ Limitations
- Less effective without Palo Alto products (firewalls, WildFire, etc.).
- Expensive for complete coverage.
🔗 More info: Cortex XDR
Need help? Contact our experts for a personalized audit and secure your business!
In-depth Comparison of Threat Detection Tools
Tool | Solution Type | Strengths | Weaknesses | Client Type | Pricing | Management Complexity | AI/Machine Learning |
---|---|---|---|---|---|---|---|
Microsoft Sentinel | Cloud-Native SIEM | Integration with Azure, Scalability, SOAR Automation | Dependence on Microsoft Ecosystem | Large Enterprises & SMBs using Microsoft | 💰💰 (Consumption-based subscription) | Easy (intuitive interface) | ✅ (Advanced detection, automation) |
Splunk Enterprise Security | SIEM & SOAR | Powerful search engine, Advanced analytics | High cost, requires complex setup | Large enterprises, MSSPs, SOCs | 💰💰💰 (Volume-based log pricing) | Complex (requires expertise) | ✅ (Event correlation, machine learning) |
Elastic Security | Open-Source SIEM | Open-source, customizable, flexible | High maintenance, requires dedicated infrastructure | Tech companies, startups, DevOps | 💰 (Free with paid options) | Complex (self-hosting required) | ⚠️ (Basic, requires advanced configurations) |
Darktrace | NDR (Network Detection & Response) | Behavioral detection, automated threat response | False positives, high cost | Critical sectors (finance, healthcare, defense) | 💰💰💰 (Annual license) | Medium (continuous learning but requires oversight) | ✅ (Advanced behavioral analysis) |
IBM QRadar | SIEM | Excellent event correlation engine, suitable for large infrastructures | Complex deployment and management | Large enterprises & SOCs | 💰💰💰 (High cost) | Difficult (long deployment time) | ✅ (AI for event correlation and log analysis) |
Vectra AI | NDR | Network threat detection specialist, high automation | Focused mainly on network (may require a SIEM for integration) | SOCs, businesses with critical infrastructures | 💰💰 (Pricing by user or appliance) | Medium (smooth interface, but needs calibration) | ✅ (Network detection powered by ML) |
Cortex XDR | XDR (Extended Detection & Response) | Multi-vector threat correlation, integration with Palo Alto | Less effective without Palo Alto firewalls | Large enterprises, Palo Alto clients | 💰💰💰 (Annual license) | Medium (centralized management but requires fine-tuning) | ✅ (Automated log and alert analysis) |
Note for writing: The comparative table is well-built, but it could be even more readable by adding: ✅ A “Ideal Use Case” column to summarize in one line the typical company that would benefit most from each solution.
✅ A rating on ease of integration to highlight which tool is the most plug & play.
Which Solution is Best Suited for Your Business?
Why Choose Microsoft Sentinel (Cloud-Native SIEM with Integrated SOAR)?
- Excellent integration with Microsoft 365 and Azure, providing advanced monitoring and automation.
- Powerful artificial intelligence engine, reducing false positives and improving event correlation.
🎯 Who Should Use It?
- Ideal for businesses already using the Microsoft ecosystem and looking for a scalable cloud-native solution.
⚠️ Limitations
- Less effective for multi-cloud businesses that are not predominantly on Azure.
🔗 More info: Microsoft Sentinel
Why Choose Splunk Enterprise Security (Advanced SIEM & SOAR for High Log Volumes)?
- Massive log management capability, with high performance for large data volumes.
- Excellent threat correlation, suitable for large SOC and MSSP infrastructures.
🎯 Who Should Use It?
- Large enterprises and MSSPs (Managed Security Service Providers) requiring a premium SIEM solution.
⚠️ Limitations
- High cost, especially for large infrastructures handling billions of logs.
- Steep learning curve, requiring cybersecurity experts.
🔗 More info : Splunk Enterprise Security
Why Choose Elastic Security (Open-Source SIEM Based on ELK Stack)?
- Open-source and flexible solution, ideal for companies seeking an affordable alternative to commercial SIEMs.
- Advanced customization with Kibana and Elasticsearch, allowing detailed log monitoring.
🎯 Who Should Use It?
- Tech companies and DevOps teams capable of managing an open-source infrastructure.
⚠️ Limitations
- No native SOAR support and incident response automation.
- Requires technical expertise for configuration and optimization.
🔗 More info : Elastic Security
Why Choose Darktrace (AI-powered Anomaly Detection and Cyber Threats)?
- Advanced behavioral detection, identifying anomalies in real-time without relying on known signatures.
- Antigena: Autonomous response module that can block threats without human intervention.
🎯 Who Should Use It?
- Companies sensitive to cybersecurity, needing a proactive and autonomous solution.
⚠️ Limitations
Numerous false positives, requiring rule adjustments.
Expensive solution, mainly suited for large enterprises.
🔗 More info : Darktrace
Why Choose IBM QRadar (Powerful SIEM with AI for Event Correlation)?
- One of the most advanced SIEMs, known for its ability to analyze and correlate events at scale.
- Integration with Watson AI, enhancing threat investigation.
🎯 Who Should Use It?
- Large enterprises with a structured SOC, requiring advanced incident management.
⚠️ Limitations
- Complex deployment and management, requiring technical expertise.
- High cost, especially for licenses including SOAR.
🔗 More info : IBM QRadar
Vectra AI (NDR Specialist – Network Detection & Response)
- Real-time network traffic monitoring, detecting threats before data exfiltration.
- Analysis of lateral movements and internal threats.
🎯 Who Should Use It?
- Companies with a strong need for network monitoring, especially those managing critical infrastructures.
⚠️ Limitations
- Only NDR functionality, often requiring a SIEM for integration.
- Less effective in cloud-only environments without internal network traffic to monitor.
🔗 More info : Vectra AI
Why Choose Cortex XDR (Palo Alto Networks) (Multi-vector Threat Management)?
- Multi-vector threat correlation (endpoints, network, cloud), offering complete threat visibility.
- Advanced response automation, with integrated SOAR playbooks.
🎯 Who Should Use It?
- Clients already equipped with Palo Alto solutions, seeking a unified detection and response solution.
⚠️ Limitations
- Less effective without Palo Alto firewalls, requiring a dedicated ecosystem.
- High cost for full coverage.
🔗 More info : Cortex XDR
In Summary, Before Choosing Your SIEM or XDR Solution, Ask Yourself These Questions
- Are your teams ready to manage a complex SIEM, or do you need a more automated solution?
- Does your budget allow for investment in a premium tool like Splunk or
- IBM QRadar, or are you looking for an open-source alternative like Elastic Security?
- Do you already use a Microsoft ecosystem, or do you prefer an independent solution?
Need help choosing? Schedule a meeting with our experts.
Fictional Examples of Issues and Possible Solutions
Example 1: A Small to Medium Business (SMB) Targeted by Phishing Attacks
Context
A medium-sized business (SMB) in the services sector is regularly targeted by sophisticated phishing campaigns. Some fraudulent emails manage to bypass anti-spam filters, resulting in user account compromises and access to sensitive information.
Challenge
- Lack of visibility into successful phishing attempts.
- Absence of correlation between incidents, making it difficult to identify repeated attacks.
- Low automatic response capability, allowing attackers time to exploit compromised accounts.
Proposed Solution: Microsoft Sentinel
- Advanced email monitoring by integrating Microsoft Sentinel with Microsoft Defender for Office 365.
- User login behavioral analysis to detect suspicious activities.
- Automated incident response: Automatic blocking of compromised accounts and strengthening multi-factor authentication.
Expected Result
- Detection time reduced to just seconds through event correlation.
- Compromised accounts blocked in under 5 minutes, preventing further exploitation.
- Improved employee awareness through attack reports and real-time alerts.
Example 2: A Large Company Facing a Ransomware Attack
Context
An industrial company experiences a ransomware attack: critical files on a server are progressively encrypted. The SOC team detects abnormal behaviors but does not know the source of the infection or how to stop it quickly.
Challenge
- Excessive log volume for rapid manual analysis.
- Difficulty identifying the point of entry and the mode of attack propagation.
- Need for a quick response to avoid total network paralysis.
Proposed Solution: Splunk Enterprise Security
- Real-time analysis of network and endpoint logs to identify the source of the infection.
- Event correlation of suspicious activities (unauthorized access attempts, data exfiltration).
- Deployment of a SOAR Playbook to automatically isolate infected machines.
Expected Result
- Attack detection in under 10 minutes after the first file encryption.
- Automatic blocking of compromised machines in under 30 minutes, stopping the ransomware from spreading.
- Identification of the attack’s entry point in under an hour, allowing the patching of the vulnerability and preventing future intrusions.
Example 3: An E-Commerce Website Targeted by DDoS Attacks and Malicious Bots
Context
An e-commerce site observes a sudden increase in traffic: thousands of simultaneous requests cause slowdowns, impacting the user experience and resulting in lost revenue.
Challenge
- Distinguish legitimate traffic from malicious bots.
- React in real-time to minimize customer impact.
- Analyze attack patterns to adjust countermeasures.
Proposed Solution: Darktrace
- Behavioral analysis of web traffic to differentiate legitimate customers from bots.
- Proactive detection of abnormal behaviors (repeated fraudulent purchases, excessive requests).
- Real-time response with Antigena: blocking suspicious IPs.
Expected Result
- Mitigation of malicious traffic in under 5 minutes, with no impact on legitimate users.
- Prevention of service interruptions, ensuring business continuity.
- Reduction of fraud attempts by blocking bots at the source.
Example 4: A Bank Facing Internal Fraud
Context
A financial institution detects unusual transactions made by employees with access to sensitive databases. The security team suspects privilege abuse, but without tangible evidence, they cannot act.
Challenge
- Detect suspicious behaviors without disrupting operations.
- Analyze access histories to identify privilege abuses.
- Implement strict controls without alerting the fraudsters.
Proposed Solution: Vectra AI + IBM QRadar
- Advanced monitoring of sensitive data access with IBM QRadar.
- Detection of behavioral anomalies (excessive client file access, mass file exports).
- Implementation of discreet alerts and automatic blocking to stop suspicious transactions.
Expected Result
- Detection of abnormal access in under 15 minutes.
- Immediate blocking of fraudulent transactions, preventing financial losses.
- Better traceability and enhanced audits to prevent further abuses.
Example 5: A Tech Company Looking for an Open-Source Solution
Context
A cloud computing startup wants to secure its DevOps infrastructures but has a limited budget. It is searching for an open-source SIEM that can integrate with its existing technology stack.
Challenge
- Find an economical and flexible solution.
- Adapt to an evolving DevOps infrastructure.
- Automate incident response without incurring additional costs.
Proposed Solution: Elastic Security
- Deploy Elastic Security to centralize logs and detect anomalies.
- Create customized Kibana dashboards to monitor suspicious activities in real-time.
- Automate incident responses using custom scripts and APIs.
Expected Result
- Quick setup with no licensing costs.
- Easy integration with existing DevOps tools.
- Continuous log monitoring and rapid anomaly detection.
Why Are These Scenarios Relevant?
These fictional cases illustrate several types of threats and the adapted solutions:
- External attacks: phishing, ransomware, DDoS.
- Internal threats: fraud, privilege abuse.
- Specific needs: open-source solutions, DevOps infrastructures.
Each company has its unique constraints, and choosing the right solution depends on:
- Technological environment (Microsoft, open-source, multicloud, etc.).
- Level of automation needed.
- Ability to manage a SIEM or XDR internally.
In the next section, we will explore how to effectively implement these solutions and optimize their integration into your cybersecurity strategy.
Facing similar challenges? Schedule a meeting with our cybersecurity experts!
Practical Guide for Implementation: Key Steps for Integrating These Tools
Implementing a SIEM or AI-based threat detection tool requires a structured approach to ensure its effectiveness. Improper integration can result in false positives, slow operations, and high costs without real security benefits.
This section guides you through the essential steps for a successful deployment and offers the best practices to maximize the value of your cybersecurity solution.
1. Audit of Existing Systems
Before implementing any solution, it’s essential to evaluate the current state of your cybersecurity to:
- Identify vulnerabilities: analyze past incidents, potential attack vectors, and existing detection gaps.
- Evaluate the compatibility of existing tools with a new SIEM/XDR/NDR solution.
- Set clear objectives: reduce detection time, automate responses, improve event correlation, etc.
Example: A company using Microsoft 365 might choose Microsoft Sentinel to take advantage of its native integration, while a multi-cloud stack company could prefer Splunk or Elastic Security.
2. Selecting the Right Tools
The choice of solution should be based on several criteria:
- Type of environment: On-premise, cloud, hybrid?
- Log analysis capability: Manageable volume and processing speed.
- Automation & SOAR: Integration with incident response playbooks.
- Budget & pricing model: Pricing based on log consumption (Sentinel, Splunk) or an open-source model (Elastic Security).
Example: A company handling a large volume of real-time logs and requiring a cloud-native SIEM might opt for Microsoft Sentinel, while a small business with a limited budget could prioritize Elastic Security.
3. Setup and Configuration
- Initial deployment: On-site, cloud, or hybrid installation.
- Integration with existing systems: Connection with firewalls, XDR solutions (e.g., Defender, Cortex XDR), log servers, and endpoints.
- Customization of rules and alerts: Define alert thresholds to avoid overloading SOC analysts.
Example: A company deploying IBM QRadar must set up advanced correlation rules to avoid an explosion of irrelevant alerts.
4. Team Training
A cybersecurity solution is ineffective without a trained team to use it. It’s crucial to:
- Train SOC teams on analyzing alerts and event correlations.
- Raise employee awareness on new security procedures (e.g., phishing attack response).
- Develop a rapid response strategy in case of critical alerts.
Example: After implementing Darktrace, a company must teach its analysts to handle false positives generated by behavioral AI analysis.
5. Ongoing Monitoring and Continuous Improvement
Once the tool is in place, it is essential to evolve it:
- Adjust detection algorithms to limit false positives and improve the relevance of alerts.
- Analyze post-detection incidents to refine correlation rules.
- Conduct regular tests to validate the system’s effectiveness.
Example: A company using Vectra AI can refine network detection models by adjusting anomaly detection thresholds for each network segment.
Mini Guide of Best Practices: The 5 Keys to Optimal Cyber Threat Detection
After exploring SIEM, XDR, and NDR solutions, as well as the best strategies for implementing these tools, here’s a quick summary of the essential best practices to strengthen your company’s cybersecurity.
1. Automate Incident Responses as Much as Possible
- Using SOAR (Security Orchestration, Automation and Response) allows you to isolate an infected machine, block a compromised account, or execute remediation actions without human intervention.
- Why? Reduce response time and SOC analyst workload.
2. Correlate Events to Reduce False Positives
- A SIEM combined with a Threat Intelligence base (MITRE ATT&CK, VirusTotal, IBM X-Force) helps avoid unnecessary alerts and prioritize critical incidents.
- Why? Improve accuracy and efficiency in identifying true threats.
3. Monitor in Real-Time
- Constant monitoring ensures that security incidents are detected and addressed quickly.
- Why? Mitigate downtime and data loss risks.
4. Use Behavioral Analysis Tools
- Behavioral detection tools, such as Darktrace or Vectra AI, are essential for detecting anomalous activities that might go unnoticed in traditional detection models.
- Why? Proactively catch emerging threats.
5. Continuously Improve Your Strategy
- Review and adjust detection methods and playbooks to stay ahead of new threats.
- Why? Adapt to evolving attack techniques and stay resilient.
Next Step: Audit and Action Plan
Threat detection is not only about choosing a SIEM or XDR tool. Its effectiveness relies on a combination of technologies, automated processes, and best human practices.
What’s your plan going forward? Evaluate your infrastructure, test your current defenses, and determine the solution that best fits your needs.
At Castelis, we are Microsoft partners and specialize in optimizing SIEM/XDR solutions. We can assist you in the selection, implementation, and optimization of your SOC and cybersecurity solutions.
📌 Need a cybersecurity audit? Contact us for a personalized evaluation.
🔗 Discover our complete analysis of Microsoft Sentinel and possible alternatives here.
Voir plus de Actualités
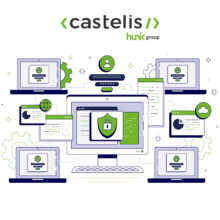
Everything you need to know about the NIS2 Directive: requirements, sanctions and compliance solutions
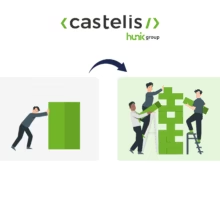
Why Adopt a Microservices Architecture? A Guide for CIOs and IT Services
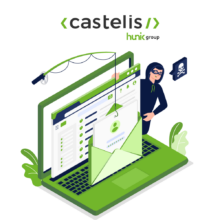
Area 1 Security: preventing and protecting against phishing attacks with Cloudflare
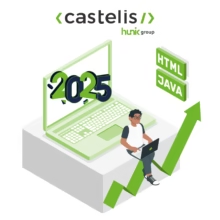